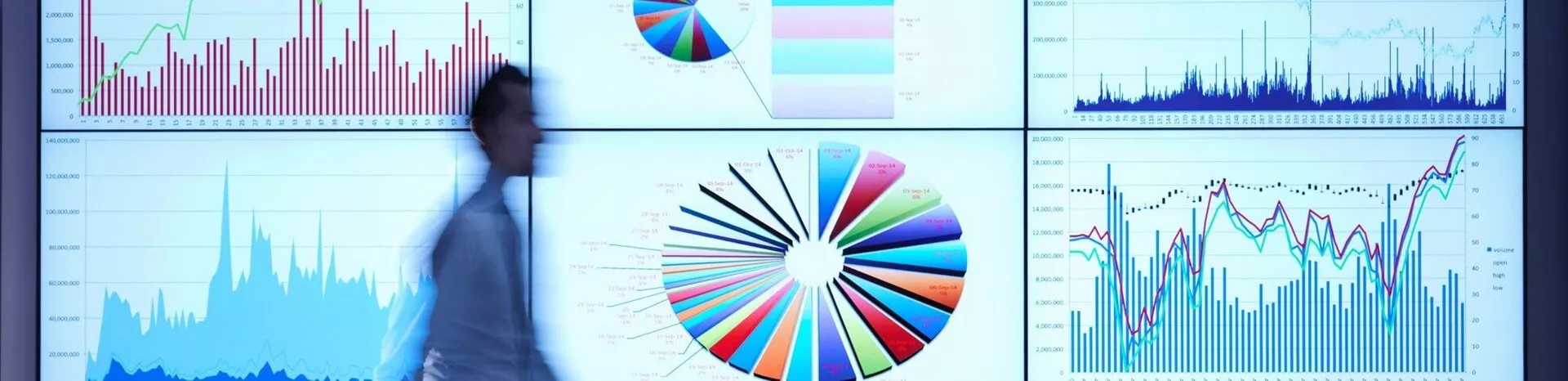
Steering and monitoring data: a performance challenge
As technology progresses in the fund custody and administration business, it has become essential for all those involved in the investment industry to seek greater control over the data required for the day-to-day running and management of their activities.
The relevance of choices in outsourcing strategies, risk management and the production of regulatory reports in line with regulators' expectations are at stake, as is, of course,the measurement of the performance of their funds in order to compare them with those of other market players.
Indeed, it is quite clear that data management currently faces multiple challenges:
Unlike market information, not all data needed for back-office processing is up to date or accessible in a timely manner (portfolio, cash account). Moreover, it cannot be shared easily, as its use is subject to the contractual documents from which it was obtained.
Apart from information structured by protocols such as SWIFT or FIX, there is a lot of unstructured, free-format data that is difficult to consolidate.
For alternative investment funds and unlisted assets (e.g. private equity and real estate), there is a need to manage a lot of additional and unstructured documentation.
When registering clients (investors or institutions), KYC1 processes require the verification of documents that are rarely homogeneous (identity documents, forms specific to each country or market).
The fund-knowledge documentation (prospectus, etc.) comes in as many formats as there are issuers or asset management companies, each of which is keen to offer the most appealing and most explicit document.
The volume of data managed daily is increasing exponentially, with new formats (podcasts, videos, tweets) also raising the level of complexity of each operation. By way of illustration, and to take a particularly current topic, ESG2 information is a good example of the growing complexity of data management and control.
At a time when the search for efficiency and productivity has never been more important in investment management, it is thus becoming increasingly difficult to control data flows and stocks. The data management role must therefore support the implementation of solutions such as SGSS' integrated suite of front-to-back services, CrossWise (processes, ad-hoc tools).
In this respect, most software publishers and technology partners will tend to provide solutions on the container, but rarely on the content. Consequently, cloud or file transfer technologies have made it possible to increase data storage capacities ("big data" environment) and to share them. But under no circumstances do these solutions make it possible to really control the management of this data to create value.
It is therefore essential to devise complementary solutions to:
Improve the quality of data (reliability, updating, source certification), and for this it is possible to rely on standardised reference systems. In this respect, the use of an IBOR3 greatly facilitates the maintenance of a centralised position in real time.
Offer solutions for data injection and input control, in order to ensure that a flow is complete, and that data is correct. STP4 flows between asset management teams and their partners are thus necessary for trading platforms, but also for the entire processing chain of middle or back office, and of course for liquidity or counterparty risk monitoring needs.
Facilitate the reconciliation of this data by structuring it (using data models and reconciliation solutions such as matching).
Add modules to generate additional value (benchmark, extrapolation), and for this the addition of Artificial Intelligence is a useful complement to our environments through automatic classification or segmentation (via machine learning, with or without supervision). Visual data recognition solutions (data visualisation) now make it possible to identify market trends or alerts on abnormal values (fraud and operational risk management). Therefore, predictive models have become very powerful when the volume of quality data is sufficient.
Easily edit management reporting, including management comments, and in so doing, help steer activities and monitor management.
In conclusion, good data management involves all processing chains and players and offers considerable challenges in terms of processing efficiency improvement on the one hand and project developmentsupport on the other. In this respect, asset servicers’ missions should also be able to move more and more towards data management. And among the solutions and tools available, both for monitoring and for steering performance, service providers are developing new models and algorithms and testing them in order to help their clientscontrol their information assets as well as possible. These models can, for example, be used to determine groups of data (segmentation, clustering), to define trends (linear regression calculation) or to find abnormal values or missing data in a series, or to identify "potential" duplicates that will then have to be checked/corrected by back officers.
With its range of solutions based on the SG Markets client platform and front-to-back outsourcing offerings such as CrossWise, SGSS supports its clients in the transformation of their organisation and supports them in improving their data management.